Researchers and engineers at Algoryx and Umeå University present the first successful implementation of a reinforcement learning controlled forestry machine. The results are presented at the International Conference on Intelligent Robots and Systems (IROS 2021).
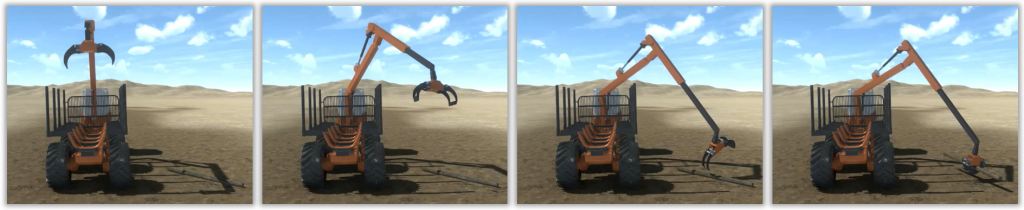
The study uses deep reinforcement learning to automate the grasping motion of a forestry crane manipulator grasping logs in a simulated environment.
Instead of being explicitly programmed to perform the loading task, the system learns the task by practicing repeatedly in simulated environments, receiving rewards for succeeding with the task, moving energy efficiently, and not colliding with the surroundings. To avoid guiding the crane to the log with very complex reward structures, it is given a large reward when it has succeeded with grasping the logs. A challenge is that the task involves many different actions that must be coordinated with the environment’s complex dynamics until a successful grasp is achieved. This makes learning very hard, something called the sparse reward problem.
– We solve this using a curriculum learning approach, says presenter and first author Jennifer Andersson. The system first learns to grasp the logs in simple situations, and the logs are then gradually placed at more difficult locations. This way, the crane becomes better and better at grasping logs it wasn’t able to grasp at the beginning of training.
Forest machines are large and heavy. They operate in rough unstructured environments and a substantial part of the training must be done in a simulated environment. The alternative is unsafe, impractical, and expensive. In a simulated environment, it is also easier to test how the system reacts to new situations and what it has really learned.
– We see evidence that the machine learning controller has learned important parts of the physics, says Martin Servin at Umeå University. It has learned to avoid actions that induce large oscillations but also to exploit the sway of the grapple for fast and robust grasping. Like a skilled human operator does.
The study was made possible using Algoryx’ physics engine AGX Dynamics in Unity3D with the machine learning tool ML-Agents. A 3D model of the concept forwarder Xt28 was provided by the company Extractor AB. More results can be expected in the near future through the research program Mistra Digital Forest, where the research team at Umeå University participates.
– The study is a first step of applying deep reinforcement learning to this problem and several challenges remain, says Kenneth Bodin, CEO at Algoryx. We are excited to see the rapid pace at which this technology develops and we are eager to help organizations realizing this on real machines.
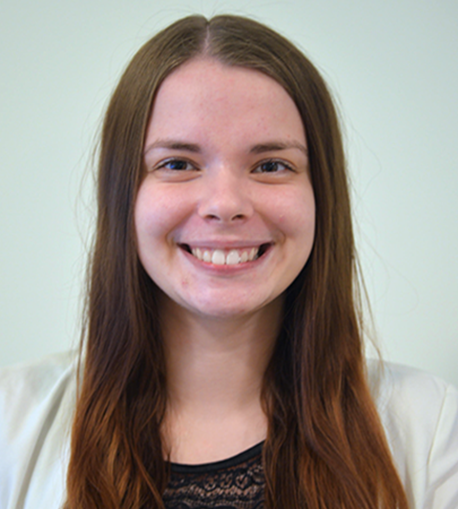
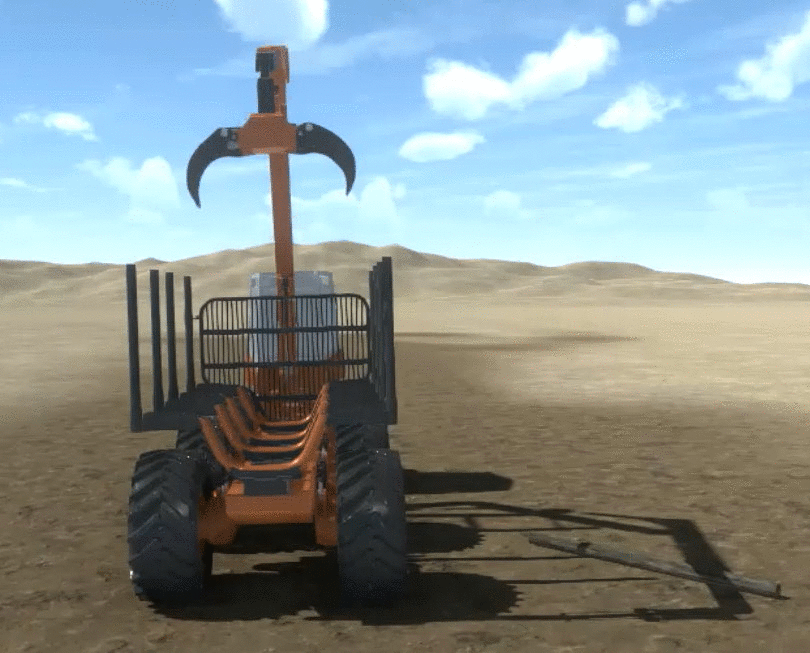
A video recording of the presentation at IROS 2021 is available here.
J. Andersson, K. Bodin, D. Lindmark, M. Servin, and E. Wallin. Reinforcement Learning Control of a Forestry Crane Manipulator. IEEE/RSJ International Conference on Intelligent Robots and Systems (IROS 2021), Sep. 27-Oct. 1st, 2021, Prague, Czech Republic (2021). arXiv:2103.02315 [videos]