Automatic control of a wheel loader doing bucket filling can be solved using deep reinforcement learning. This was recently demonstrated in a simulator environment by a team from Algoryx, Epiroc, and Umeå University. The scientific paper was recently published in the journal Machines.
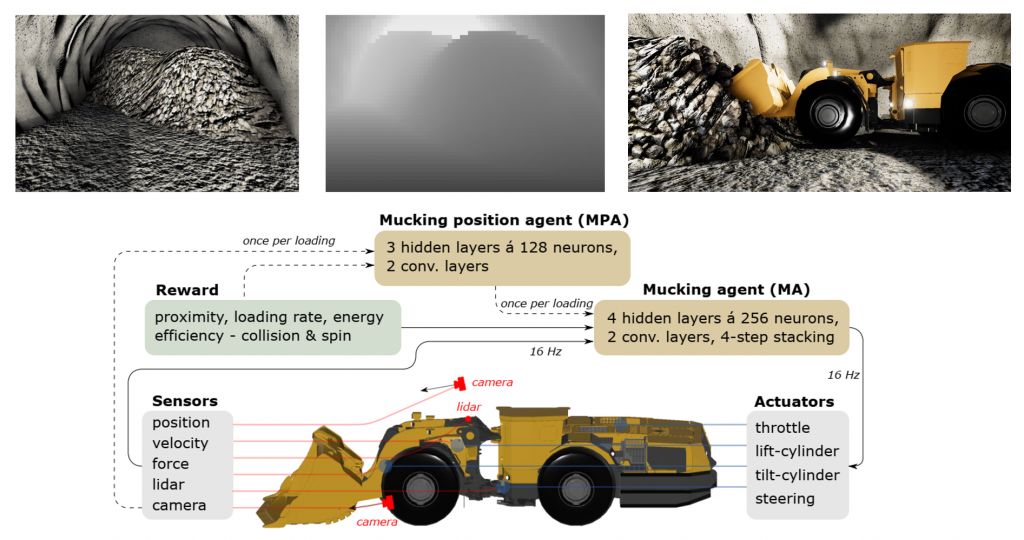
In underground mines, specialized wheel loaders (LHD) are used for loading fragmented rock, hauling the material through narrow tunnels, and dumping it for further transport on trucks or hoist systems. The loading task remains a challenge to automate. To fill the bucket efficiently, an operator, or an artificial intelligent agent, must coordinate the forward drive, steering, and the bucket’s lift and tilt in response to visual cues, and the perceived force resistance and vehicle motion.
To test whether deep reinforcement learning is a viable approach to automating this task, a simulated environment was constructed with a 3D multibody model of a loader and narrow drift with virtual piles of fragmented rocks using AGX Dynamics for Unity and ML-Agents. The vehicle, an Epiroc Scooptram ST18, was equipped with a depth camera, and force and motion sensors. A multiagent system was trained using the reinforcement learning algorithm Soft-Actor-Critic and a curriculum setup.
First, a mucking position agent learned to predict the most efficient position to dig into differently shaped piles. Next, a mucking agent was trained on how to control the vehicle to fill the bucket at a selected dig position. Finally, the agents were trained together. The agent’s policies were represented by a feedforward neural network with convolutional layers for handling the high-dimensional observation data. The rewards the agents learn to maximize were designed to maximize bucket filling with minimal energy consumption and avoiding collisions and wheel slip.
The learned policies were able to adapt to previously unseen pile shapes, achieving a success rate of 99% with an average bucket load of 13.2 tonnes. The productivity and energy efficiency was better than the values reported for human operators in the scientific literature, but it remains to test if these results transfer to the physical environment.
Further details are found in the article that is available under open access in the special issue “Design and Control of Advanced Mechatronics Systems” in the scientific journal Machines:
S. Backman, D. Lindmark, K. Bodin, M. Servin, J. Mörk, and H. Löfgren. Continuous control of an underground loader using deep reinforcement learning. Machines 9(10): 216 (2021) . doi.org/10.3390/machines9100216 [video]
This project was funded in part by VINNOVA (grant id 2019-04832) in the Integrated Test Environment for the Mining Industry (SMIG) project.
For more information about the project and how to use AGX Dynamics in your own projects, please contact Algoryx Sales.